The AI Institute of the Medical University hosts research groups focusing on various areas of artificial intelligence / machine learning methodology and applications in biology and medicine.
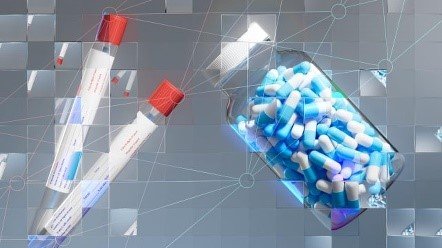
Machine Learning in Biomedicine
(Christoph Bock)
Our research combines machine learning with genome technology, single-cell biology, and cell engineering. We are working on personalized therapies for cancer and immune diseases. We are part of the European Laboratory for Learning and Intelligent Systems (ELLIS.eu) and the Human Cell Atlas. More information: https://bocklab.org.
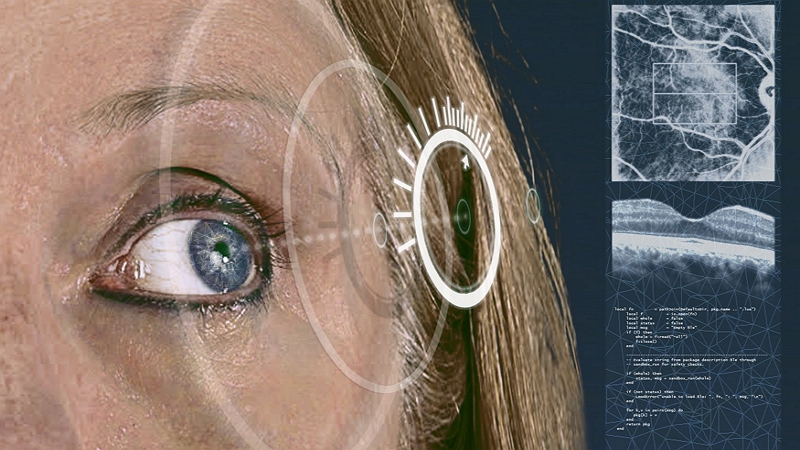
Medical Image Computing
(Hrvoje Bogunović)
Our research is dedicated to advancing the machine/deep learning methods for medical image analysis in order to enable trustworthy clinical decision support systems and propel clinical research. A particular focus is on the interpretation of retinal imaging data, and predicting disease progression from longitudinal imaging. More information: https://www.meduniwien.ac.at/cdair
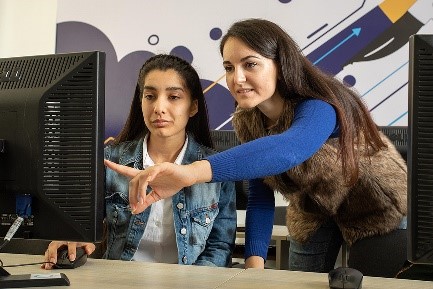
Machine Learning for Prediction and Signal Processing
(Georg Dorffner)
Our focus is two-fold: (a) Classical machine learning applied to patient data to predict diseases or their outcomes, (b) Deep learning and other methods applied to signal processing, in particular to the interpretation of EEG and other electrophysiological signals, such as, for instance the automated scoring of polysomnograms in sleep medicine.
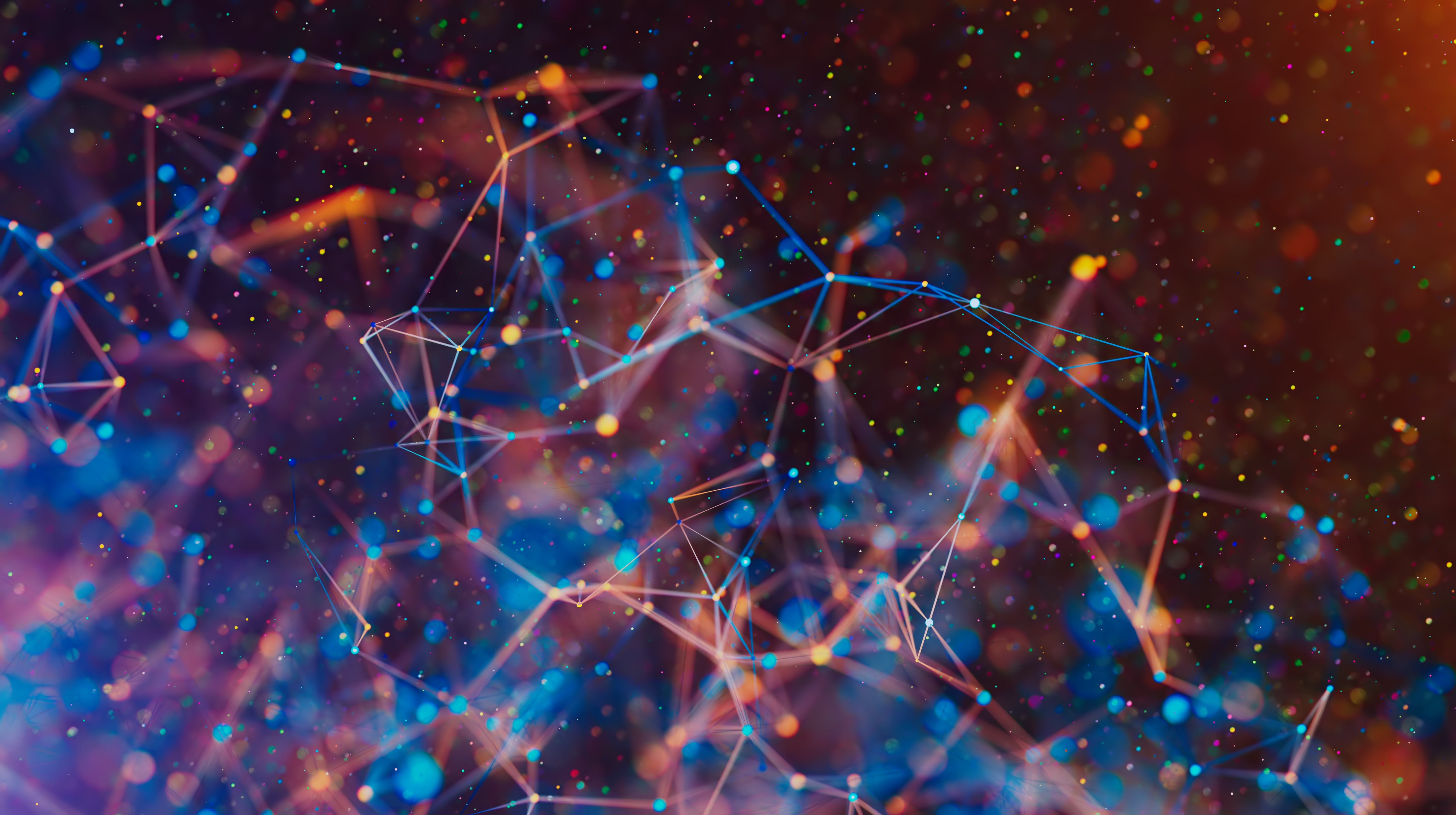
AI in Systems Biology
(David S. Fischer)
We develop mechanistic machine learning models to study cellular systems in human disease using omics experiments. Specifically, we design models that contextualize these large datasets within the corpus of existing molecular biology knowledge. Inspired by systems biology, we iterate between modeling and experimental validation. More information: https://ai4biomedicine.org
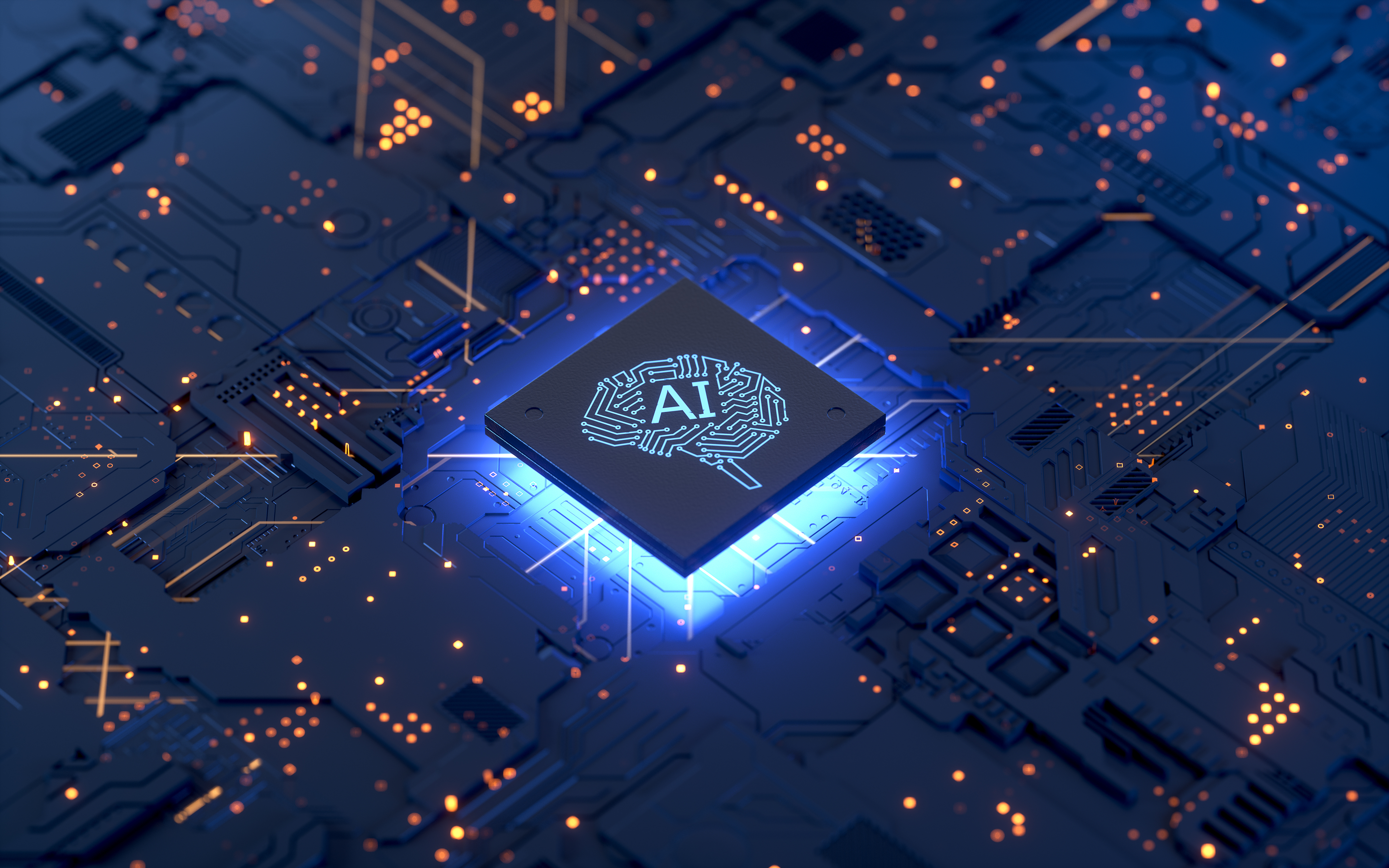
Dynamics of Neural Systems
(Adam Gosztolai)
Our research aims to understand how the brain and AI systems solve computational challenges through the dynamical activity of neural populations. We use machine learning, geometry and dynamical systems theory to bridge the gap between biological and artificial systems and to develop methods that benefit clinical and broader scientific applications.
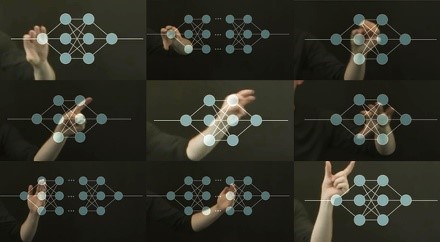
Trustworthy AI
(Matthias Samwald)
AI systems are evolving rapidly and are poised to revolutionize biomedical research and clinical practice. But with increasing capabilities come new challenges and risks. Our research group is dedicated to the mission of ensuring that advanced AI systems are robust, interpretable and beneficial. More information: https://samwald.info/
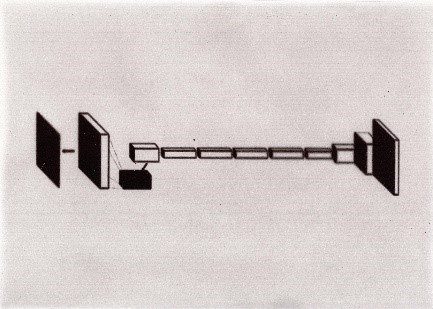
Deep Learning Methods & Applications
(<position to be filled>)
We are recruiting a group leader / assistant professor with research focus on deep learning methodology and applications in medicine. This future research group will pursue methods development in machine learning (e.g., interpretability, causality, generative models), together with prototypical applications in medicine or biomedical research.